Deep Machine Learning for Quantifying Protein Dynamics from High-Speed Atomic Force Microscopy Data​
​
December 11, 2020
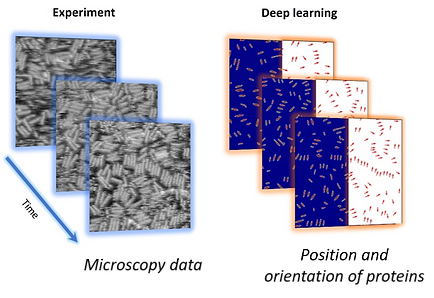
A deep learning (DL) network trained on a single labeled video frame is used to predict position and orientations of proteins in the entire movie (~600 frames) in a matter of seconds.
Scientific Achievement
A deep learning (DL) based workflow reveals the dynamics of protein self-assembly on inorganic substrates at the particle-by-particle level.​
​
Significance and Impact
This work establishes the power of DL for the analysis of complex self-organization processes from advanced characterization data and provides insight into the fundamental mechanisms that underpin the system's behavior.
​
Research Details
-
The dynamics of self-assembly of a de novo designed protein were visualized using video-rate atomic force microscopy.
-
A DL network trained on a single frame was used for rapid conversion of the entire AFM movie into proteins position and orientation.
-
Possible classes of protein behaviors were identified, along with transition probabilities.